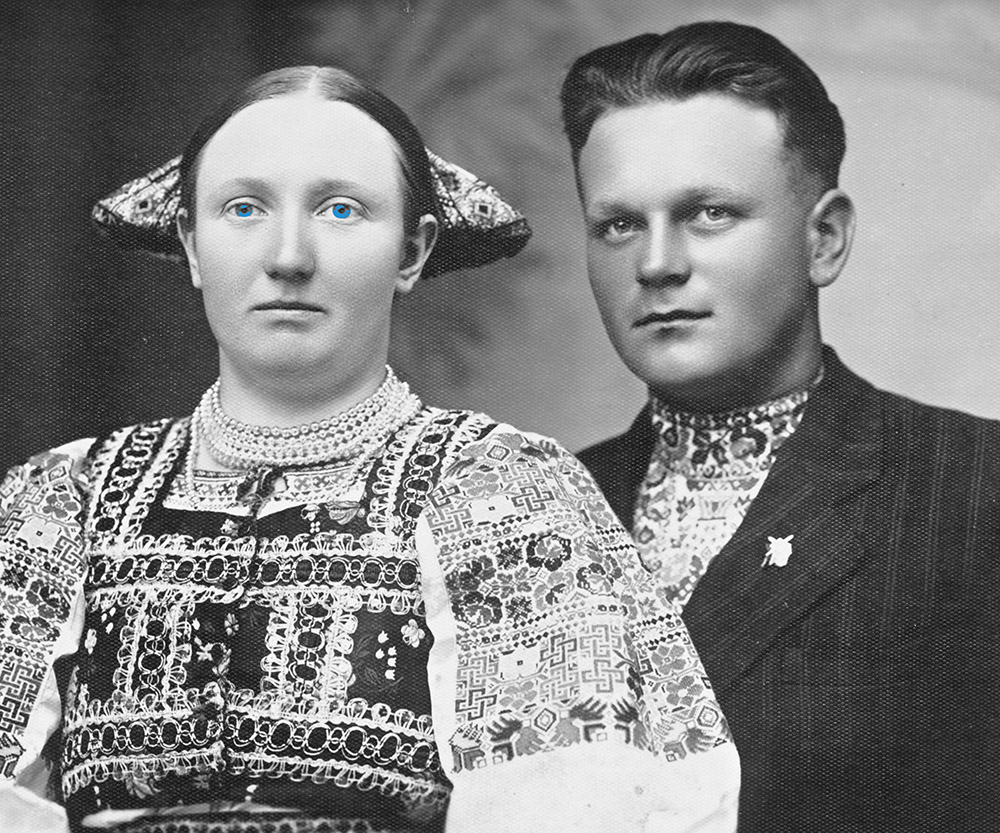
Research Echoes #2
Research Update, 21/04
But what are the values of the new generation?
Research never stops, especially in our beloved field of Deep Learning. Since staying up-to-date with this research is a necessary (but not sufficient) condition for quality, we must remain vigilant and continue to provide updates on the latest developments. Last time, we discussed chatGPT (and the media-technical uproar that accompanies it). Today, we present two different topics that have seen very innovative work in recent times: data augmentation through diffusion models, and MetaAI's Segment Anything, which has quickly become a new reference.
Synthetic data, data augmentation & diffusion models
Since Rombach et al.'s Stable Diffusion in June last year, we can only observe the explosion of these generative approaches, which have, in a few months, almost made our good old Generative Adversarial Networks disappear from the radar. Even NVIDIA, the former promoter of GANs (and author of StyleGANs), has converted to this iterative approach.
A form of application has emerged in recent weeks and seems particularly interesting for us humble engineers looking for new tools. I'm talking about the use of these models to enrich a dataset, either through a data augmentation approach or through a synthetic data generation approach. Two recent works are worth considering:
Effective Data Augmentation With Diffusion Models [https://arxiv.org/abs/2302.07944] by Trabucco et al. is very interesting in that it proposes a specialization of data augmentation (aiming to make a model more robust against simple variance) using diffusion models. This approach allows generating a new image from an image where potentially the main subject or the background will be modified. The new image will retain the photorealism characteristic of diffusion but will add a very strong beneficial variance against overfitting. Note that the authors discuss in detail the balance between synthetic and real data, and they propose in particular an approach to object-centric augmentation that can be very qualitative for instance segmentation problems.
Why are these works of interest to us? Synthetic data is already identified as an important element of any Deep Learning approach. Generally, it is advised to avoid generative models like GANs, which are mainly "bias machines" (recall the problem of CycleGan hallucinating tumors in medical imaging). Moreover, since OpenAI's work on Domain Randomization [https://arxiv.org/abs/1703.06907], it is known that the most realistic data is not necessarily wanted, but rather a strong variance allowing to "blur" the target distribution by generalizing.
Why are these works interesting to us? Synthetic data is already recognized as an important element of any Deep Learningapproach. Typically, it is advisable to avoid generative models like GANs , which are often "bias machines" (recall the problem of CycleGan hallucinating tumors in medical imaging). Moreover, sinceOpenAI work on Domain Randomization [[https://arxiv.org/abs/1703.06907], it has been understood that the goal is not necessarily to have the most realistic data possible, but rather a strong variance that allows for "drowning" the target distribution by generalizing.
One of the constraints of a synthetic data generator is to be perfectly controllable in order to aim for a particular variance in the data. However, diffusion models are becoming increasingly controllable (for example, via the recently released version 1.1 of ControlNet). The photorealism added to the control capability provides a new tool that, while not recommended for every problem, could in some cases address data deficiencies in certain subjects. Therefore, we are working to analyze the opportunities of this new approach in order to offer them to you as soon as possible.
Segment Anything (Anything)
Beyond impressive results, the most significant aspect is that this work from MetaAI, consisting of both a model and a dataset, is released under an Apache license, allowing for peaceful reuse in a professional setting. The authors, Kirillov et al, offer the largest segmentation dataset to date with over one billion masks across more than eleven million images. Considering the importance of large and high-quality datasets, this point is already very important, enabling more effective pretraining on specific subjects. The authors also propose a model that, beyond being state-of-the-art on this dataset and its academic siblings, can be used in a very interesting manner.
Beyond impressive results, what's most important is that this work by MetaAI consisting of both a model and a dataset is distributed under an Apache license, allowing for peaceful reuse in a professional setting. The authors, Kirillov et al, present here the largest segmentation dataset existing, with over a billion masks across more than eleven million images. Considering the importance of large and high-quality datasets this aspect is already very significant, enabling more effective pretraining on specific topics. The authors also propose a model that, beyond being state-of-the-art on this dataset and its academic counterparts, can be used in a very interesting manner.
The model can indeed be queried on an image with three types of complementary data: one or more points of attention, a bounding box, or text. While the latter point is in line with current trends, the former is very interesting because it directly allows for envisioning deployment of the model, where a user queries the model by indicating only a few locations that will generate the output mask. This type of approach is always more interesting than global end-to-end approaches because it leaves the door open to iterative interaction with a human user.
It is also noteworthy that the model can be adapted for zero-shot transfer, addressing a new problem without specific training. The authors experiment with contour detection, proposing "interesting" objects, instance segmentation, and text-to-mask generation as direct applications.
To say that this model has shaken up the academic world is an understatement. Within weeks, several extensions have appeared. Among them :
- Inpaint Anything [https://arxiv.org/pdf/2304.06790v1.pdf] for direct application to inpainting (recreating content within an image)
- SAMM (Segment Any Medical Model) [https://arxiv.org/pdf/2304.05622v1.pdf]: an adaptation (perhaps a bit risky) to medical imaging
- Can SAM Segment Anything? When SAM Meets Camouflaged Object Detection [https://arxiv.org/pdf/2304.04709v2.pdf]: a welcome critique (as with any iteration in research) on the limits of SAM, particularly in cases of occlusions
SAM is currently being tested in our environment. While it may not adapt to all industrial problems (especially optimization issues), it does indeed appear to be a fundamental new tool in segmentation that we can offer you if appropriate.
Miscellaneous
Let's note two other recent works of interest, albeit somewhat unrelated to the themes discussed above :
- DINOv2 [https://arxiv.org/pdf/2304.07193v1.pdf]: DINO was a foundational model in the contrasting (and critiqued) family of Vision Transformers; any reader following this field won't miss new version of MetaAI
- A Method for Animating Children’s Drawings of the Human Figure [https://arxiv.org/pdf/2303.12741v2.pdf]. Encore MetaAI (promis, nous surveillons nos biais, mais MetaAI a récemment été très actif sur des travaux de qualité), cette fois ci sur l’animation automatique et charmante de dessins d’enfants. Parce que parfois, cela fait du bien de moins se prendre au sérieux 🙂